[ad_1]
The Anticipated Returns of Machine-Studying Methods
Does the funding in subtle machine studying algorithm analysis and growth repay? It is a crucial query, particularly in gentle of the growing prices associated to the R&D of such algorithms and the potential of lowering returns for some strategies developed within the extra distant previous. A latest paper by Azevedo, Hoegner, and Velikov (2023) evaluates the anticipated returns of machine learning-based buying and selling methods by contemplating transaction prices, post-publication decay, and the present excessive liquidity surroundings. The obstacles aren’t low, however analysis means that regardless of excessive turnover charges, some machine studying methods proceed to yield optimistic internet returns.
Current monetary analysis has highlighted the spectacular capability of machine studying (ML) strategies to foretell inventory returns. Research typically report exceptionally excessive annualized Sharpe ratios for ML-driven buying and selling methods, typically attaining over 5 occasions the historic market common.
Regardless of these promising outcomes, their real-world applicability stays debated. Critics level out that whereas ML fashions can exploit hard-to-arbitrage shares, their efficiency could decline as a result of excessive turnover and different financial constraints. Furthermore, real-time implementations of those methods typically present weaker efficiency, particularly when accounting for transaction prices.
The research goals to bridge this hole by assessing the anticipated returns of ML methods, contemplating the impacts of transaction prices, post-publication efficiency decay, and trendy market liquidity. By using numerous ML strategies on a dataset of 320 anomalies, the researchers discovered that though some methods underperform after prices, many nonetheless ship important returns, notably these utilizing superior fashions like Lengthy Brief-Time period Reminiscence (LSTM) networks.
These findings have vital implications for each tutorial analysis and the design of sensible funding methods. As we advance additional into the period of machine studying and AI, such research shall be important for navigating the complexities of monetary returns and gaining a deeper understanding of market dynamics.
Authors: Vitor Azevedo ; Christopher Hoegner and Mihail Velikov
Title: The Anticipated Returns on Machine-Studying Methods
Hyperlink: https://papers.ssrn.com/sol3/papers.cfm?abstract_id=4702406
Summary:
This research assesses the anticipated returns of machine learning-based anomaly buying and selling methods, accounting for transaction prices, post-publication decay, and the post-decimalization period of excessive liquidity. Opposite to claims in prior literature, extra subtle machine studying methods are worthwhile, incomes internet out-of-sample month-to-month returns of as much as 1.42%, regardless of having turnover charges exceeding 50% and deciding on some difficult-to-arbitrage shares. A buying and selling technique that employs an extended short-term reminiscence mannequin to mix anomaly traits yields a six-factor generalized (internet) alpha of 1.20% (t-stat of three.46). Whereas prevalent cost-mitigation strategies cut back turnover and prices, they don’t enhance internet anomaly efficiency. General, we doc return predictability from deep-learning fashions that can’t be defined by widespread danger elements or limits to arbitrage.
Associated paper: Reducing Returns of Machine Studying Methods – QuantPedia
As at all times, we current a number of fascinating figures and tables:


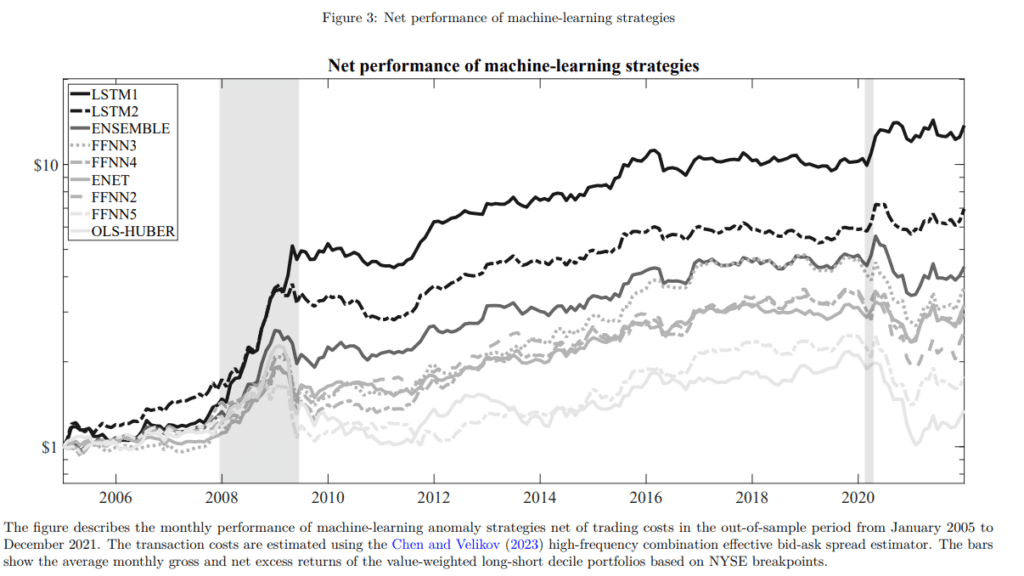
Notable quotations from the educational analysis paper:
“So far, we estimate the long-short returns of machine studying fashions with out accountingfor transaction prices. Avramov et al. (2022) argue that when transaction prices are launched,most fashions don’t present statistically important returns as a result of their efficiency largelydepends on small, illiquid, and costly shares. We check this explicitly and observe a reductionin common month-to-month returns and general monetary efficiency after accounting for the Chen andVelikov (2023) efficient bid-ask unfold estimate. Determine 2 exhibits the proportion drop in averagereturns for the 9 machine-learning methods from introducing buying and selling prices. We will observethat the discount in efficiency ranges from 13% to 40%.”
“Desk 3 and Determine 4 present the influence of the beforehand outlined mitigation approacheson our 4 lessons of mannequin architectures, specifically linear fashions, FFNNs, LSTMs, and theensemble mannequin. We present the influence of absolute variations within the internet extra return portfoliometrics and generalized FF6 alpha and relative adjustments in turnover and transaction prices. Asthe outcomes present, a lot of the cost-mitigation strategies considerably cut back turnover and, as aresult, transaction prices.“
“This lower in transaction prices, nevertheless, is just useful if it isn’t accompanied by alarger discount in gross returns. As we are able to observe in Desk 3, the typical change internet excessreturns throughout the 9 machine studying fashions is detrimental for all however one mitigation approach.This means that the drop within the gross common returns as a result of mitigation strategies morethan compensates for the decreased buying and selling prices. That is possible as a result of our testing pattern interval,which consists of the final twenty years, is marked by greater liquidity and considerably lowertrading prices post-decimalization (Chordia et al., 2014; Chen and Velikov, 2023). The onlytechnique that appears to marginally enhance the web common returns throughout the 9 machinelearning methods is the two-month holding interval. Not surprisingly, the inventory universe filtershave a smaller influence on turnover however the same influence on transaction prices, as they intention toreduce the burden of high-cost shares. Nevertheless, the change in internet extra returns for thesemethods is equally detrimental.”
Are you searching for extra methods to examine? Join our publication or go to our Weblog or Screener.
Do you need to study extra about Quantpedia Premium service? Test how Quantpedia works, our mission and Premium pricing provide.
Do you need to study extra about Quantpedia Professional service? Test its description, watch movies, evaluate reporting capabilities and go to our pricing provide.
Are you searching for historic knowledge or backtesting platforms? Test our checklist of Algo Buying and selling Reductions.
Or comply with us on:
Fb Group, Fb Web page, Twitter, Linkedin, Medium or Youtube
Share onLinkedInTwitterFacebookSeek advice from a pal
[ad_2]
Source link